How to Get a Paper Accepted
A case study
In 2019, I submitted a paper that was rejected with review scores 2.5, 3, 3. One week later, I resubmitted it with minor changes, and it was accepted with scores 4, 4.5, 4.5.01
This essay is about those minor changes.
As an unexpected bonus to getting the paper accepted, such tweaks significantly improve the actual science contribution, as weâll see at the end.
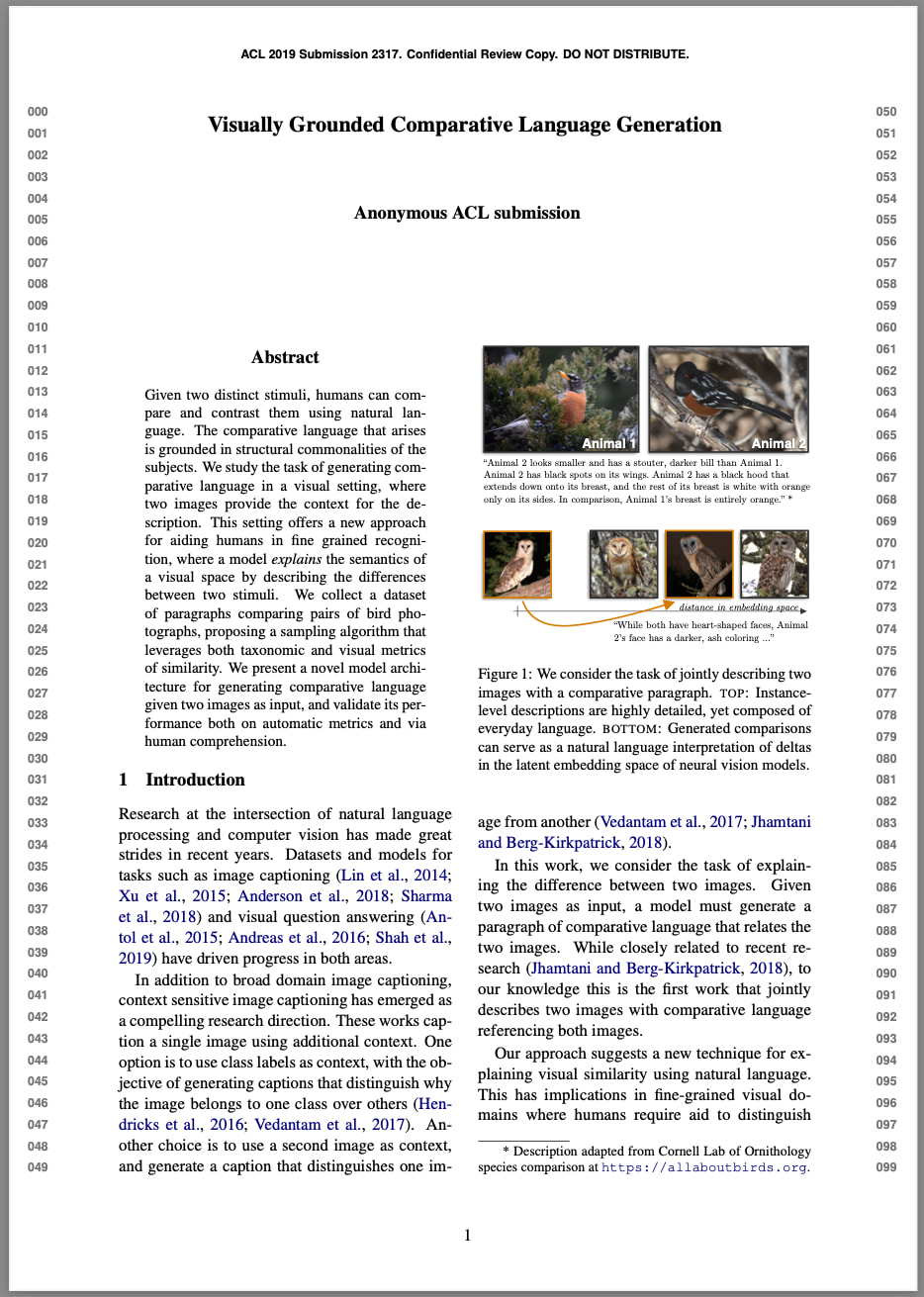
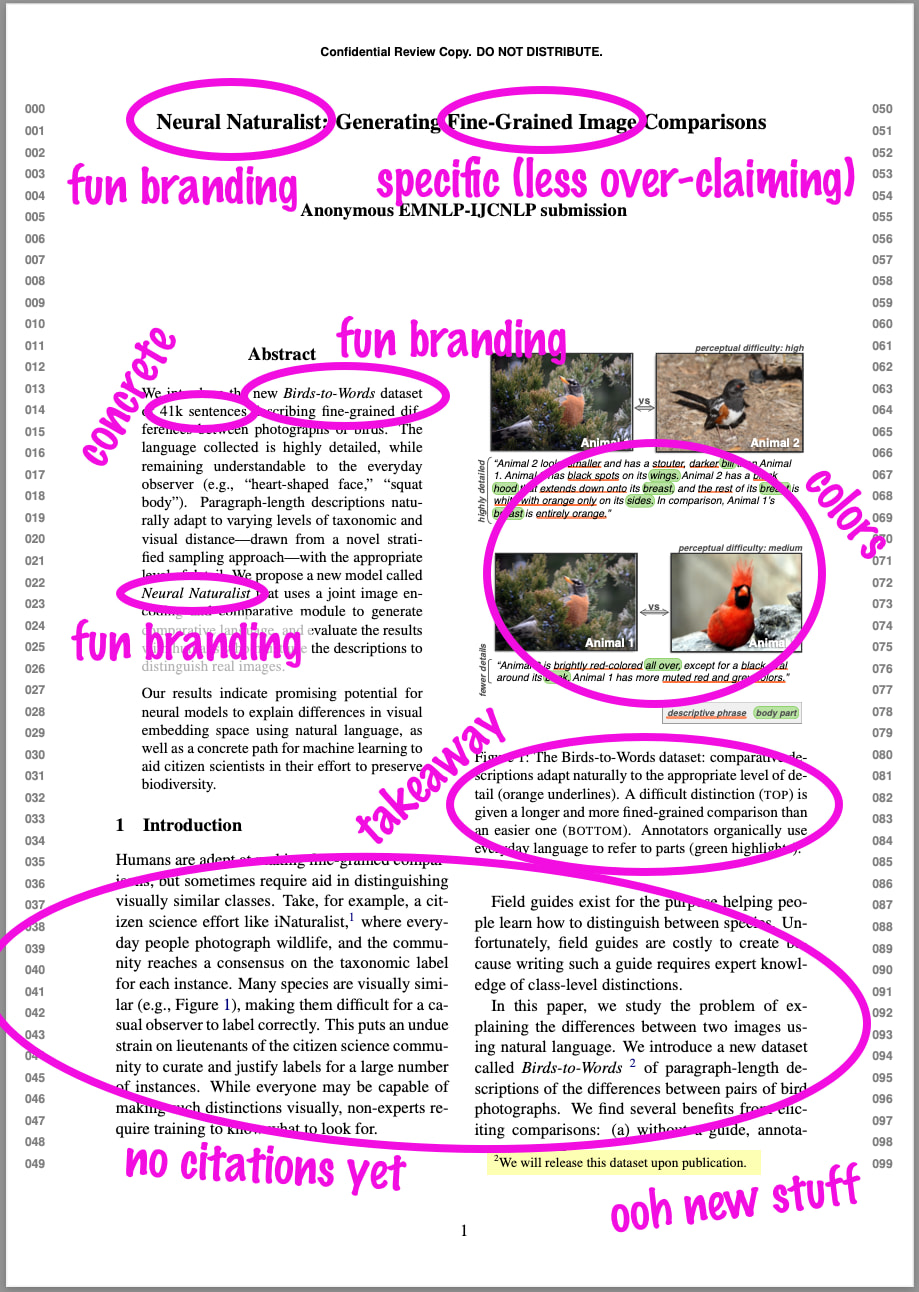
Top: Left: Rejected page 1. Bottom: Right: Accepted page 1.
Prerequisite: Do good work
Iâm assuming that you are doing quality research, and you want it to be published to further your career. You need to get past the gatekeeping reviewers. In other words, please use this process for good and not evil.02
Think in Reverse
At the highest level, I approach getting a paper accepted by thinking in reverse:
- Think of all the reasons a reviewer might reject your paper
- Avoid everything in 1.
This isnât totally trivial, because a big reason is âI didnât get itâ (which is internalized as âitâs boringâ and then spelled âlow impactâ in the review). But revising to avoid rejection is still easier than doing the research itself. Clear writing is difficult but doable.
The more obvious rejection reasons have to do with completeness: âyou didnât compare against method X.â But those are often used as objective crutches to justify a gut decision based on lack of clarity. So we must work on both.
Optimizing Clarity and Completeness
For this paper, everything we improved likely applies to all papers:
- Title specificity
- Branding
- Task clarity
- Takeaways in figure captions
- Writing (abstract / intro / conclusion): top-down, boring, focused on past work, vague, implying incremental and failed â specific, interesting, tension/release cycles, difficulty and benefit-motivated, with unique hooks, arguing value
- Differentiation vs othersâ work
- Highlighting model03 interest
- Baselines
- Ablations
- Pickier examples
These changes can be sorted into two buckets:
- Completeness â Baselines, ablations (often also: human evaluations)
- Clarity â Everything else.
Now Iâll walk through all the changes in that longer list. By no coincidence, theyâre all visible in (a) page 1, or (b) a figure or table.
Page 1: ~75% of Your Paper
A paper has four parts:
- Title
- Figure 1
- Abstract
- Rest of the paper
Spend equal time on all four of these.
â Me misquoting04 Jitendra Malik quoting Don Geman
Around 75% of a paperâs quality is established on page 1. The title, Figure 1, abstract, and visible introduction are all there. Itâs like a bookâs cover.
Here are both page 1s again for reference:
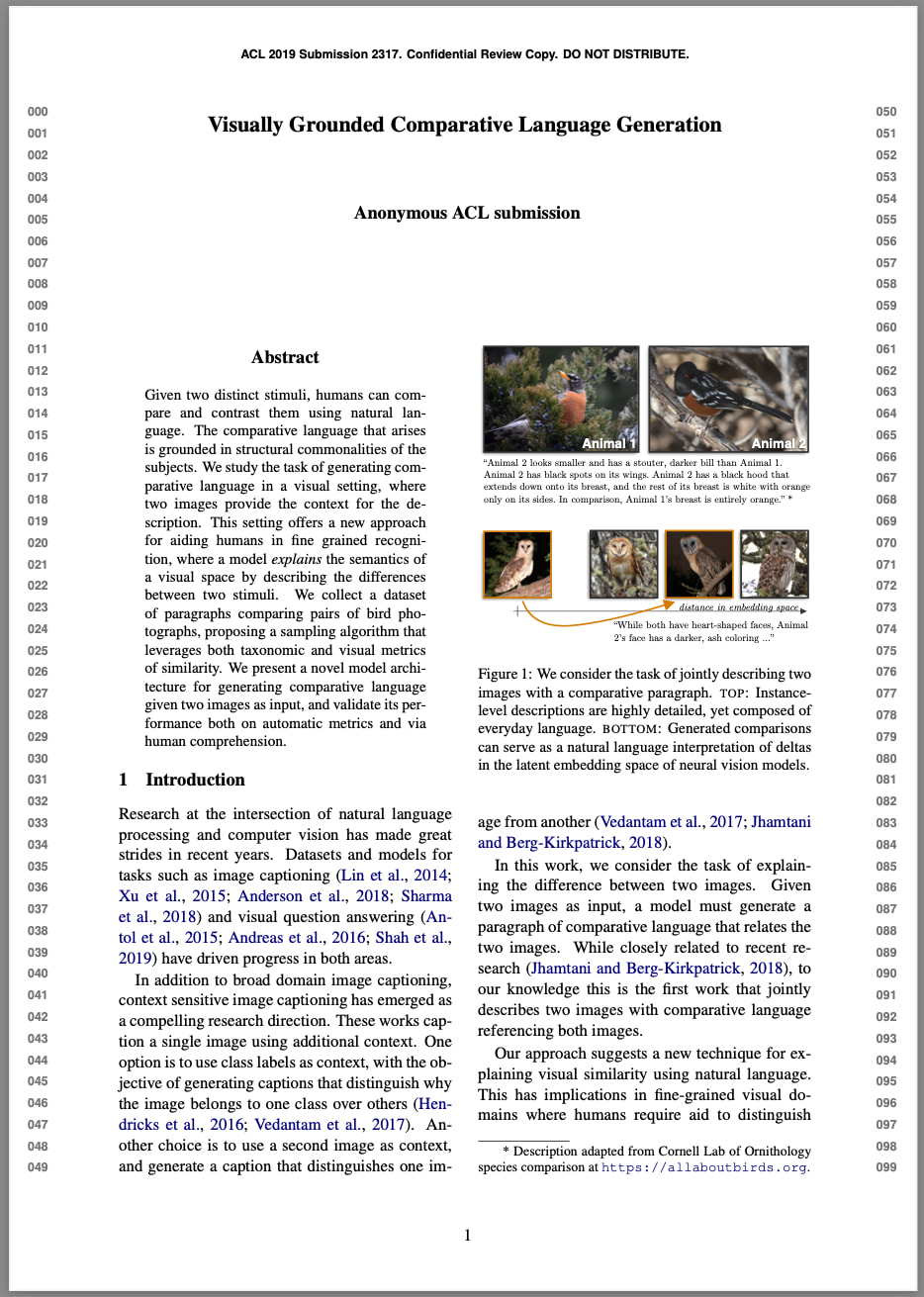
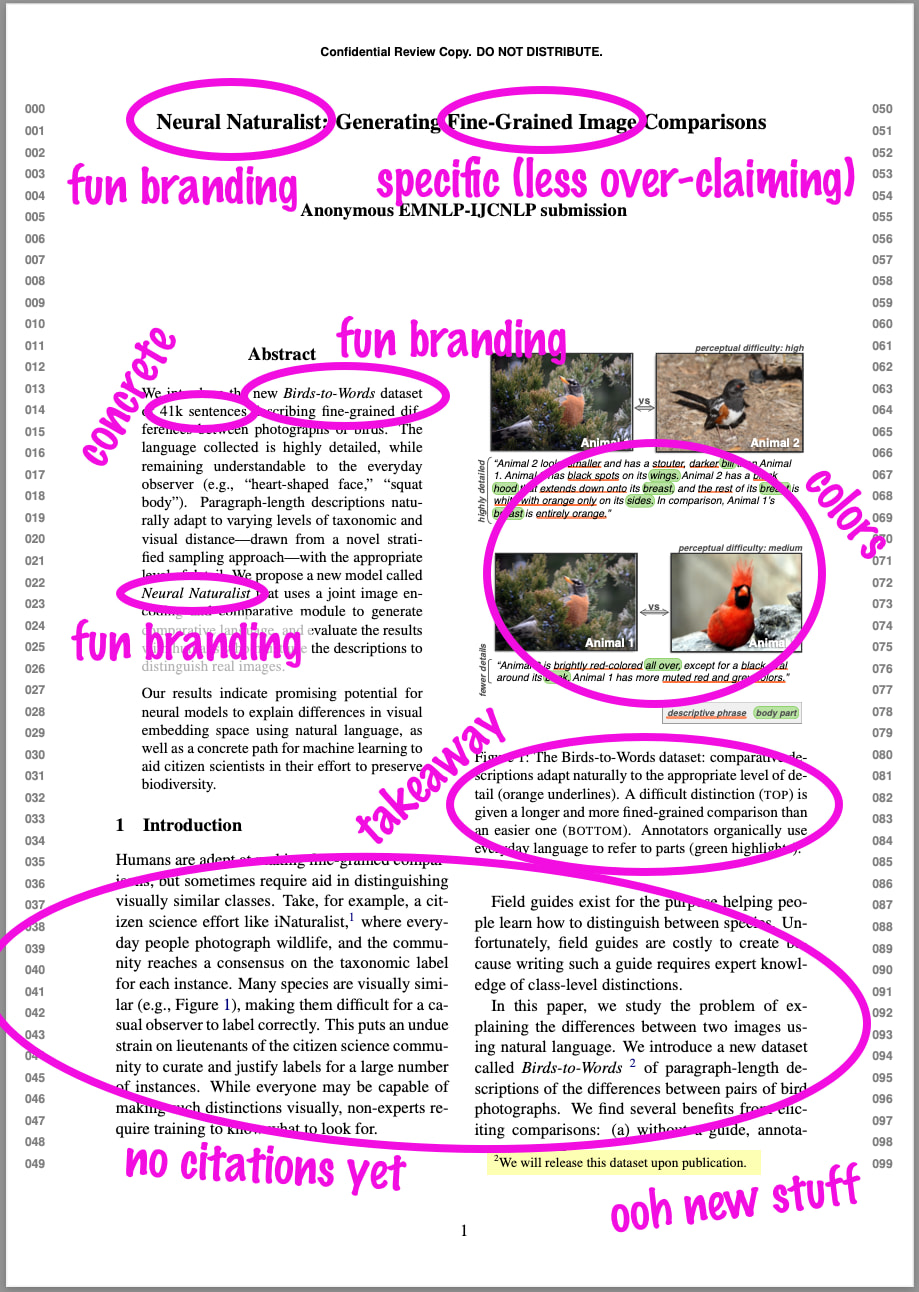
Top: Left: Rejected page 1. Bottom: Right: Accepted page 1. Takeaway: A birdâs-eye (ahem) view of the paper should draw readers in with a specific and compelling title and Figure 1, and lead with concrete contributions rather than top-down exposition and related work comparisons.
Weâll break down each aspect in the following sections. But first, itâs worth thinking about whether a quick scan draws a reader in:
- Is the Figure 1 visually appealing? (Iâm guessing eyes go to the picture first. At least mine do.)
- Is the title intriguing?
- Are there any interesting terms (bolded or italicized)?
- Is the introduction full of citations? (Itâs OK if so, but itâs a different vibe, and probably harder to pull offâmore in line with an opinion piece.)
Title: Specific and Memorable
Rejected: Visually Grounded Comparative Language Generation â too general. Any work that uses pictures and generates comparisons could use this title. I picked this title because I thought it argued for the generality of the method. But a too-general title is off-putting because it comes across as over-claiming. And a big part of our method does rely on our domain: we specifically use a biological taxonomy to create our dataset.
Accepted: Neural Naturalist: Generating Fine-grained Image Comparisons â specific and memorable. In addition to branding (more next), naturalist establishes the domain, and fine-grained narrows the task. Skeptical academics appreciate the clarity of saying what you did. The title is fully unique to our work.05
Branding: Maybe Do It
I used to dislike branding in papers. It felt presumptuous to claim a proper noun for your research paper and to expect readers to memorize it. And many of the names sound corny.
Now, while I still often feel a pang of annoyance, it is outweighed by the recognition that itâs much easier to remember and discuss concepts which have a name. Neural naturalist or Birds-to-Words instead of âour 2019 EMNLP paper about generating comparative image captionsâŚâ
That said, I still dislike throwaway namesâthose with no conceptual link, or which donât feel earned. I donât think every paper needs one. But I think it helped for this paper.
Figure 1: Screamingly Obvious Value
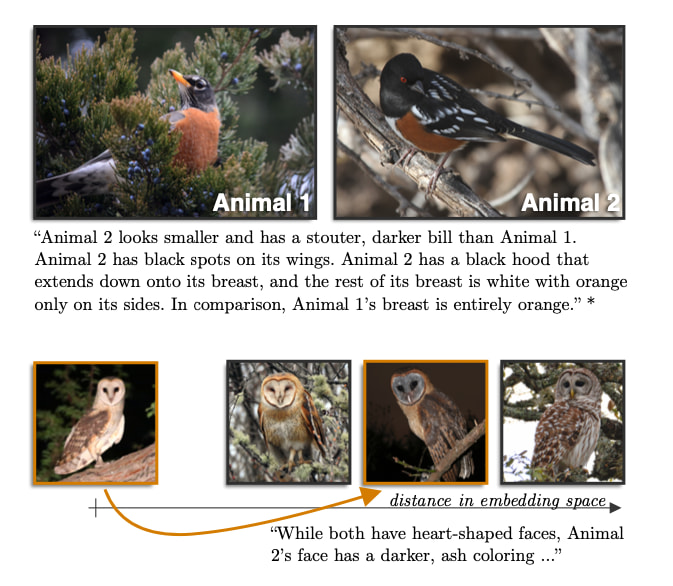
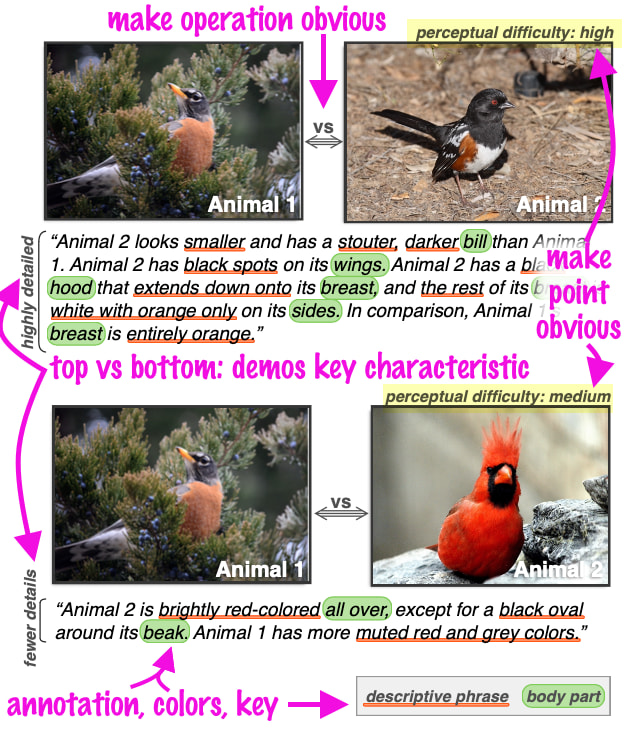
Top: Left: Rejected Figure 1. Bottom: Right: Accepted Figure 1. Takeaway: After a spark of visual appeal, Figure 1 must make the paperâs key contribution screamingly obvious and demonstrate its value.
A Figure 1 should
- draw readers in
- clearly demonstrate both what the work does and its value, and
- be comprehensible without the caption.
The old Figure 1 showed two separate comparisons, but the link between them wasnât clear. The bottom row just all look like owls to a non-expert. And the descriptions are long and boring.
The new Figure 1 makes the worksâ focus explicit by anchoring with the same left image, and labeling each comparison with a perceptual difficulty (âhighâ vs âmediumâ). It annotates the operation (âvsâ = comparison) and the result (âhighly detailedâ vs âfewer detailsâ). At this point, the paperâs mechanics and unique characteristic has been established: we use different language to compare things based on how similar they look. Finally, to make the long descriptions more approachable and interesting, weâve highlighted two components (features and parts, with orange underlines and green bubbles).
A problem with making Figure 1sâand describing your research in generalâis that you know so much about it, itâs impossible to mentally model what itâd be like to learn about your work for the first time. Spending time away from your work is extremely helpful here, if possible. I think I benefitted by having the conference review period (a month or two?) away from the paper, so I could come back to it with fresh eyes and rethink how best to illustrate it.
Iâve written about Figure 1s before. Even at the peak of my Figure 1 game, it was normal to make ten drafts before submitting.
Captions: Takeaway
The best paper-writing advice I ever got was: make the final sentence of each caption the takeaway.
This Figure 1 is so information-dense the whole caption is the takeaway. Compare vs the old caption which, has side note (red) taking nearly 1/3 of the (extremely valuable front-page) real estate!
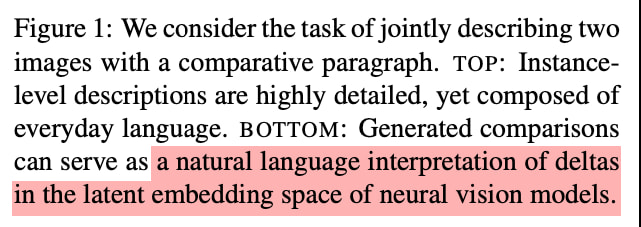
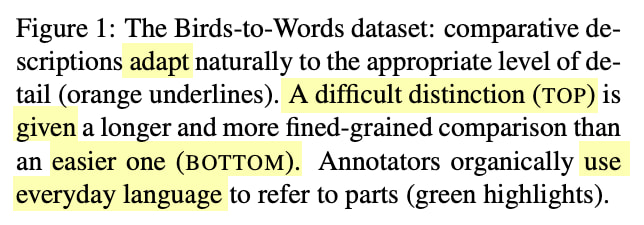
Top: Left: Rejected Fig 1 caption with a long aside (red). Bottom: Right: Accepted Fig 1 caption. Clear and direct language communicates the takeaway points (yellow). The âtakeawayâ pattern will become more obvious in future captions.
A takeaway message explains not what is literally being shown in the figure (that comes first), but what you should think about it.
It might feel strange to do this in scientific writing, because it feels like it crosses the boundary from description into interpretation. But I urge you to do it, especially for less formal fields like computer science because:
-
Youâre saving readers time trying to understand what point you are trying to make by just writing it out.06
-
With good captions, you can understand the whole paper by only looking at the figures. Many (most?) future readers will do this.
-
The scientific reader has a grain of salt mindset about everything you write anyway, so donât stress about the âinterpretationâ aspect.
If you arenât trying to prove a point, perhaps reconsider that figure.
I got even more brazen about takeaways in future papers, even writing bolded âTakeaway:â in the caption itself. E.g., check this one from Scarecrow (Dou & me et al., 2022)
This is a great example because the tableâs interpretation is so complicated that even I had forgotten what the takeaway was supposed to be a few years later, and would not have easily rediscovered it.
Abstract: Specific, Valuable, Hook
A classic mistake for a certain type of nerd is to write top-down, going from general concepts to your specific topic. This is tempting because it feels orderly and taxonomic.
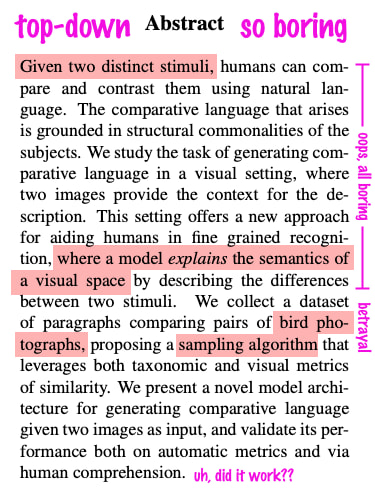
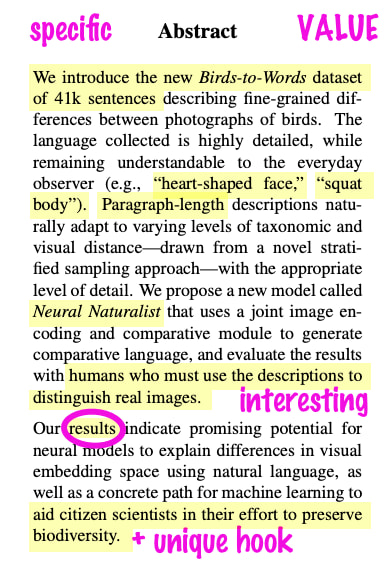
Top: Left: Rejected abstract, with top-down boring framing, an aside, and then a âbetrayalâ of scope when we reveal our actual task (red). Bottom: Right: Accepted abstract. Everything is more specific: what we study, our contributions (dataset and model), all the way to literal descriptions of specific birds and the task done in human evaluations (yellow).
But this ends up terribly. Itâs both boring and feels over-claiming.07
Instead, the revised abstract jumps into exactly what we do, why itâs interesting, a results teaser, and hint of a unique hook. Itâs not only more specific, itâs more fun and compelling to read.
You donât think your reader wants to have fun and read something compelling? Try reviewing conference papers. Enjoyable writing is like water in a desert. They wonât even realize why theyâre happy, theâll just like the paper. Read YOLOv3 and tell me you donât enjoy it.08
Intro: Judgment-Before-Scroll
Can you believe weâre still on page 1? Itâs that important.
Here weâre discussing specifically the portion of the introduction visible on page 1. Weâre optimizing for what we could call judgment-before-scroll.
My original draft was so bad itâs easy to improve. But if I could write something this bad as a 4th year PhD student, others could too.
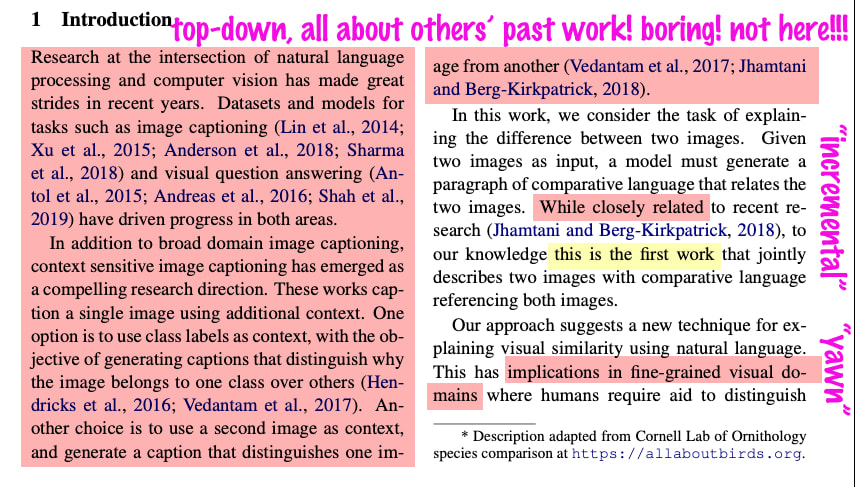
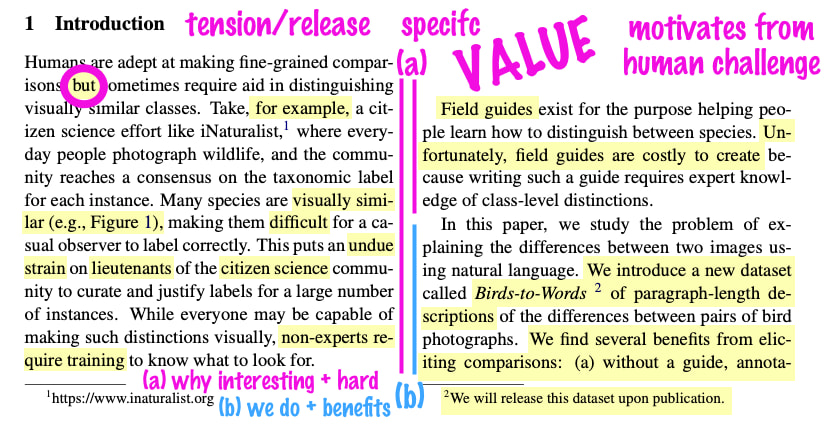
Top: Rejected page 1 intro. The problem here is familiar from the abstract: a top-down framing of the work, followed by negative implications (red). Bottom: Accepted page 1 intro. On the backbone of tension/release cycles at different resolutions, we spend the first two paragraphs setting up our task as being specific, difficult, valuable, and unique. (And I really mean each of those adjectives.) The final visible text introduces one contribution (the dataset) (yellow).
While I hesitate to sprinkle in a recommendation here because itâs (a) eighty minutes long and (b) slightly abstract, Larry McEnerneyâs talk on Effective Writing is the single best source Iâve seen on thinking about your writing. I saw it way after grad school, but I wish Iâd seen it during because I spent a lot of time blindly reverse engineering bits of it (on display in this essay). Some key points relevant here:
- All of life before your job (writing research papers counts), people (teachers) have been paid to read your writing
- Now that theyâre not, your writing must deliver value (which is often entertainment)
- High-value text poses problems with tension-filled language, articulating costs or benefits
My original introduction completely lacked any mention of a problem, and was devoid of tension. It was an orderly framing of related work which situated our contribution.
The revised one leads with the difficult problem. In addition to the larger tension/release cycle (paragraph 1+2 are problem (tension), paragraph 3 is solution (release)), there are tension/release cycles at the sentence scale (âbut,â âdifficult,â âstrain,â âwhile X, Y,â âunfortunatelyâ) which build up the stakes of the problem, i.e., the perceived value.
I didnât understand this framing (of problem, tension, value) while writing the revision. But in hindsight, itâs shockingly clear how faithfully the improved draft adheres to it.
Everything Else
After page 1, the main changes are:
- adding baselines
- adding ablations
- improving all the figures and tables
The rest of the paper is nearly identical (we also rewrote the conclusion, which Iâll show). This is great both because someone skimming the paper can enjoy all the improvements, and because it makes the rest of this essay easy for you and me.
Figure 2: Enliven and Utilize
Thereâs this complicated part of the paper called pivot-branch sampling. I was very excited about it but nobody else cared about it (I think not even my coauthors, though they were too kind to ever say.)
I had the decency to relegate most of it to the appendix, but it has to be mentioned a little bit in the body because itâs a dataset paper.
Still, the clarity just wasnât there. Figure 2 was supposed to help, but it didnât. In the revision, I added some graphics, which helps quickly get the idea across.
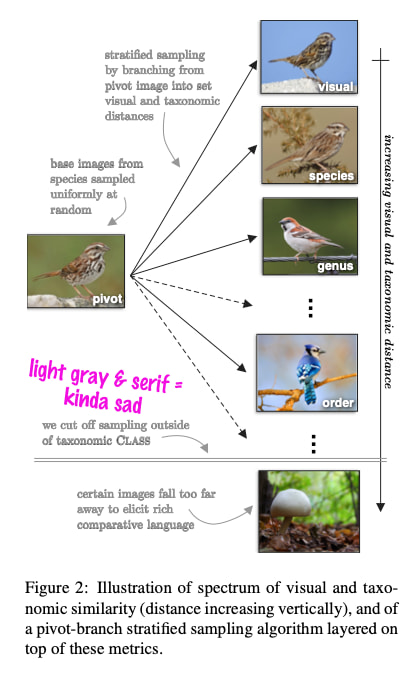
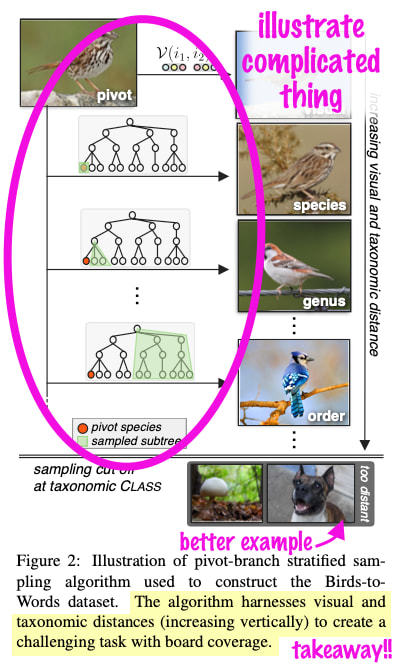
Top: Left: Rejected Figure 2. I thought lighter gray text would be nice (thereâs a design rule that you shouldnât use pure black), but it contrasted weirdly with the paperâs body text, which is rendered differently and is pure black. Bottom: Right: Accepted Figure 2. I went with a sans-serif, black text which helped the figure feel solid and distinct. And more importantly, I used the real estate to illustrate a complicated thing and has a great visual representation (the pivot-branch sampling).
Dataset Table: Invent Helpful Taxonomy
Reviewers were confused about our dataset. Why was it interesting or valuable?
I had shot myself in the foot with crappy writing that situated the contribution as incremental and marginally different (see abstract and intro sections above), but thereâs no harm in over-correcting, right?
We first introduced this tableânew in the revisionâjust to contrast example sentences from the most related datasets. This alone would have been greatâexamples are densely impactful brain magic.
But one of the biggest brain blasts I had was realizing that I could simply invent helpful axes (circled) along which to compare the datasets.
Dataset comparison table (new in accepted version). Not only are examples incredibly helpful to get a flavor of things, the taxonomy I made up helps with quantitative (ish) framing.
Inventing the dataset taxonomy helped free up my brain from imaginary rules. For example, the data citations wouldnât fit in the table without destroying the alignment. What to do? Well, I simply moved them to the caption. Can you do that? Nobody complained.
Dataset Stats: A Little Plot
A chart helps break up the visual rhythm of a paper. Plus, it can demonstrate a property thatâs otherwise hard to grasp. (Here: that we have longer text than other datasets.)
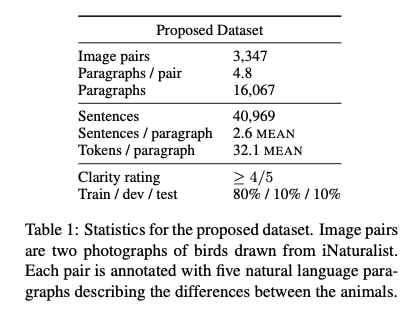
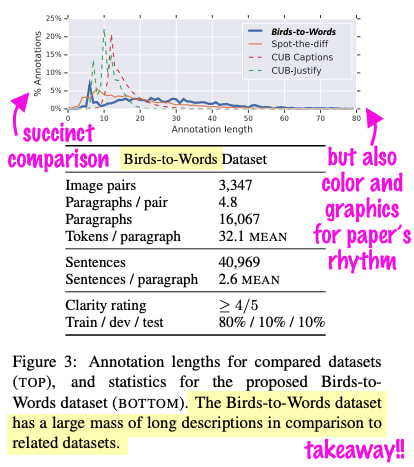
Top: Left: Rejected dataset stats. Bottom: Right: Accepted dataset stats. Critically: the takeaway message is the last sentence in the caption.
Model: Make it Shine
I had done a bad job highlighting how interesting the model was. In the revision, I not only drew out the components we ablated (yellow, red), but I used color to link them to the results table later in the paper. As a bonus, we now have warm colors (yellow, red) for the encoder and cool colors (blue, green) for the decoder.
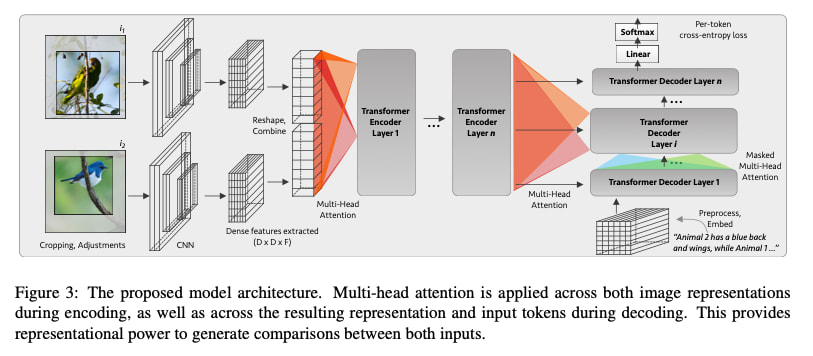
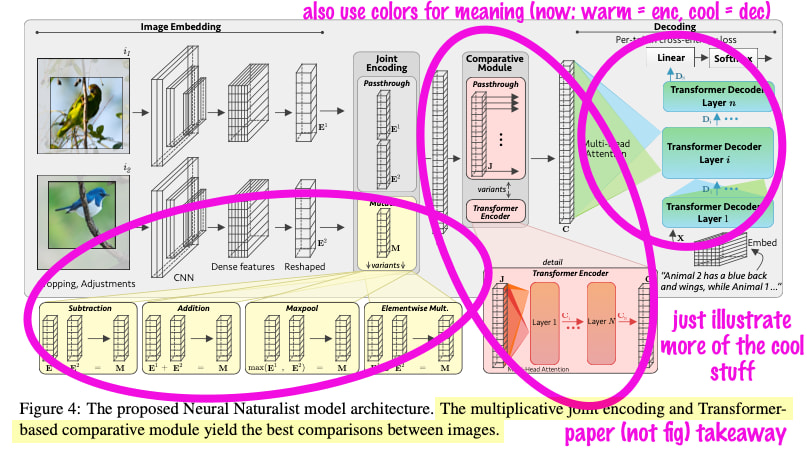
Top: Rejected model figure. Bottom: Accepted model figure. I help the reader out by telling them in advance what configuration of the model works best as the takeaway sentence of the caption. This is another good trick to remember: donât withhold information to surprise readers. They like to know early and often. I am guilty of this and itâs still a hard habit to break.
Outputs: Easy Spot to Cut
Several changes above take up more space. Where do we cut?
Fortunately, we already had a figure with an excessive number of outputs. Iâm a big fan of showing your systemâs outputs, so Iâd included nine scenarios (i.e., eighteen total photos and paragraphs). This is great, but trimming to six scenarios still leaves plenty. Plus it let us be pickier with which ones were included.09
Notice thereâs no takeaway sentence here. Rules are guidelines. If the takeaway feels belabored and out-of-place, omit it.
Results: Donât Get Rejected
This is where the thinking in reverse part comes in at full force. Think of the most common reviewer complaints and avoid them.
The easiest reasons reviewers could give to reject you were:
- lack of baselines
- lack of ablations
- lack of human evaluation
So, add those things.
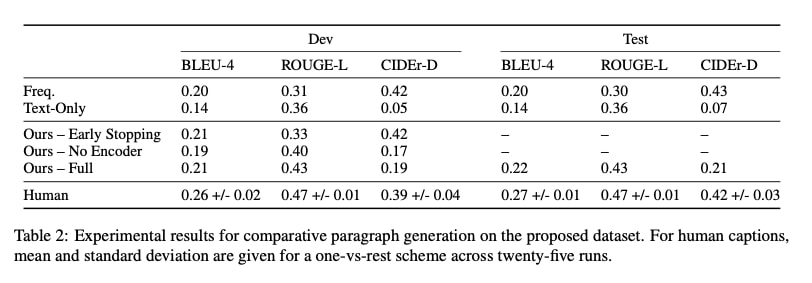
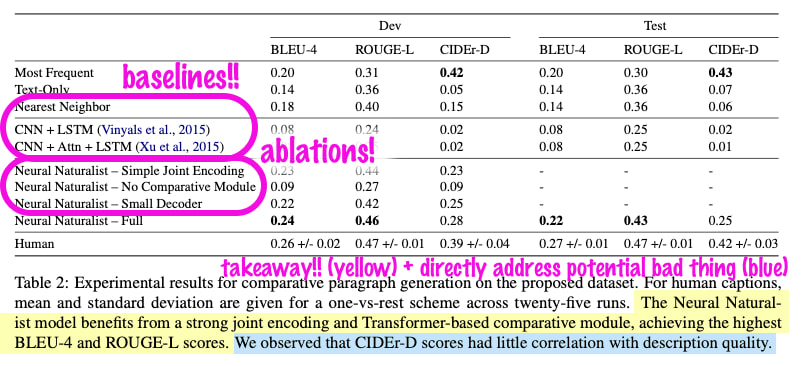
Top: Rejected results. Bottom: Accepted results. Adding baselines and ablations = good. (Also blindly bolding highest numbers. Sorry.) My favorite part: In the takeaway (yellow), we highlight and explain a weak-looking result (blue).
The baselines and ablations took relatively little work to run and probably improved the actual science contribution (more on that soon).
We already had a dream human evaluation, which is getting people to use the captions for an objective task (i.e., can you pick which animal is which?) rather than scoring them on subjective quality metrics (e.g., how fluent is the text 1â5?). No changes there.
Ablations: New
Somehow we made space for an enormous table of ablations. Running lots of ablations is a luxury of having a small dataset.10
The blind bolding of higher numbers without statistical significance tests is truly heinous, I know. I hope somebody has standardized tests that you run on output metrics by now to do this. (Just kidding, Iâm sure they havenât.)
Conclusion: A Three-Sentence Highlight Reel
To revise the conclusion, distill the advice from the abstract and introduction. Also, remove all the framing.
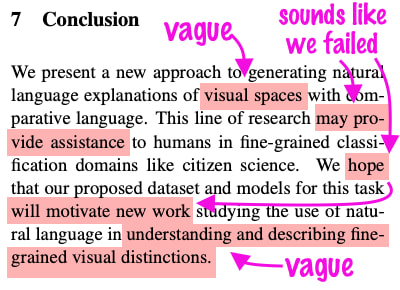
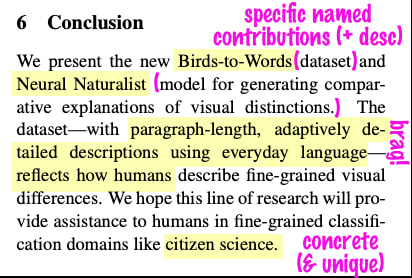
Top: Left: Rejected conclusion. Bottom: Right: Accepted conclusion. Weâre left with a concrete, three-sentence highlight reel.
Normal writing advice would say something like this: Write your conclusion using three sentences:
- What do we do?
- Why is it great?
- Why does it matter?
But check out the rejected conclusion. It (roughly) follows this structure too! The real improvement in the revision is specificity.
The Science Thing Was Improved
After making these mostly aesthetic revisions and seeing the paper accepted with such dramatically higher scores, the initial thrill wore off, and I grew more cynical of science. While we had improved the framing of our work, the core science thing we achieved was the sameâthe dataset, the model, the human evaluation, and the overall task framing itself (which is the hardest part).
Now, I believe such seemingly-surface dressings actually strengthen the underlying science thing. A lot. The primary objects of modern science are research papers. Research papers are acts of communication. Few people will actually download and use our dataset. Nobody will download and use our modelâthey canât, itâs locked inside Googleâs proprietary stack.11 But anyone who reads our paper could learn from what we did, and all the revisions to clarity and completeness improve how much they can learn per minute spent reading. (See Science 1 vs Science 2 in this essay series for a more thorough treatment of this argument.)
Aside: The idea of âmaking a reader want to read moreâ has an unexpected link to game development. Youâd think thereâd be no need for such antics in a scientific research paper, yet dull obtuse prose can scare off readers, obscure the message, and deflate the contributionâs impact. Getting readers to the endâat least of page 1âis a necessary goal to optimize for. Just so with game design and âhooks.â Games employ several hooks to draw players along, which might quickly be lumped into: stories build tension, todo lists beg completion, and ânumber goes up.â Omitting these entirely robs a game of âstickiness,â12 leading players to grow bored and stop early. In both papers and games, we must learn to make the object sufficiently engaging so that its consumer is driven to experience the bulk of our creation.
Appendix: Full PDFs
If youâd like to check out the original, raw PDFs that we submitted, theyâre available for download here. The appendices (i.e., supplementary material) are nearly identical, but Iâve also included them for completeness.
Footnotes
Review scores spanned 1â5, with 5 = âconsider for best paper,â and 3 = âweak accept.â The conferences were both of equal prestige (ACL and EMNLP respectively). Also, I use âIâ for simplicity, but as always, this was work done with coauthors. âŠď¸
But if you use it for evil itâs not a big deal either. Another ignored paper will be in a conference instead of just on Arxiv. âŠď¸
If your paper lacks a model contribution, substitute model with your key innovation. Though for us, the dataset was really the key contribution, and differentiating it was the most important part. âŠď¸
His quote had âIntroductionâ instead of âFigure 1,â but I stand by my revision. Thanks to Kenneth Marino and David Freire for finding the source of this quote. Jitendraâs talk is greatâI watched it after writing the first draft of this and couldnât believe how much overlap there was! (I never saw his talk, but someone told me about that quote.) Also, aside, donât get hung up on senior advisors thinking they actually spend as much time working on the title as you do writing the rest of the paper. Yes the title is really, really important, but they donât. Let them think they do. âŠď¸
Having now watched Jitendraâs talk (linked in the quote above), he articulates this brilliantly: the title should âevoke the key concept of the paperâ and âbe memorable.â But my favorite part: âthink about it in terms of the conditional entropy;â your title should only be able to describe your paper and no one elseâs (at a conference). âŠď¸
I must point out again that your point will be so obvious to you because itâs why you spent hours making the figure, but a new reader may barely spend enough time looking at your thing to understand what the axes are. Help them out. Even stuff like âhigher is betterâ is helpful unless completely trivial. âŠď¸
Why does it feel like it over-claims? I think because thereâs an implicit promise that if youâre talking about something, your paper is going to address it. So if youâre outlining broad swaths of a field, even if in an attempt to just situate your work, it can come across as implying that youâre contributing to this whole grand situation. (This isnât a hard ruleâsome context in the intro or related work is helpful.) âŠď¸
As with everything, there is a balance to strike here. Engaging writing and very unique hooksâe.g., having the phrases âcitizen scienceâ and âbiodiversityâ in an NLP paperâmust come as sprinkles on top of a solid contribution that appropriately engages with the expectations of the community. âŠď¸
I think the other place we saved the most space was in the qualitative analysis. I could probably write eight pages of only qualitative model analysis, so I always end up with too much in the first draft. âŠď¸
Also, being somewhere like Google. DeepMind wasnât busy with the TPUs that week so we added a bunch of flags and let them go brrr. But the dataset is so small that by the time Googleâs ancient behemoth cluster system had made a dashboard where I could see how the run was going, it had already ran over the whole training dataset (potentially many times, memory is failing me). âŠď¸
Even if it were open source, let me tell you from first-hand experience that getting someoneâs research code to run is no small feat, especially under even marginally different conditions. âŠď¸
On the other hand, leaning too hard into them and using darker patterns (like gambling mechanics, even worse with real money) causes addiction and bankruptcy. âŠď¸